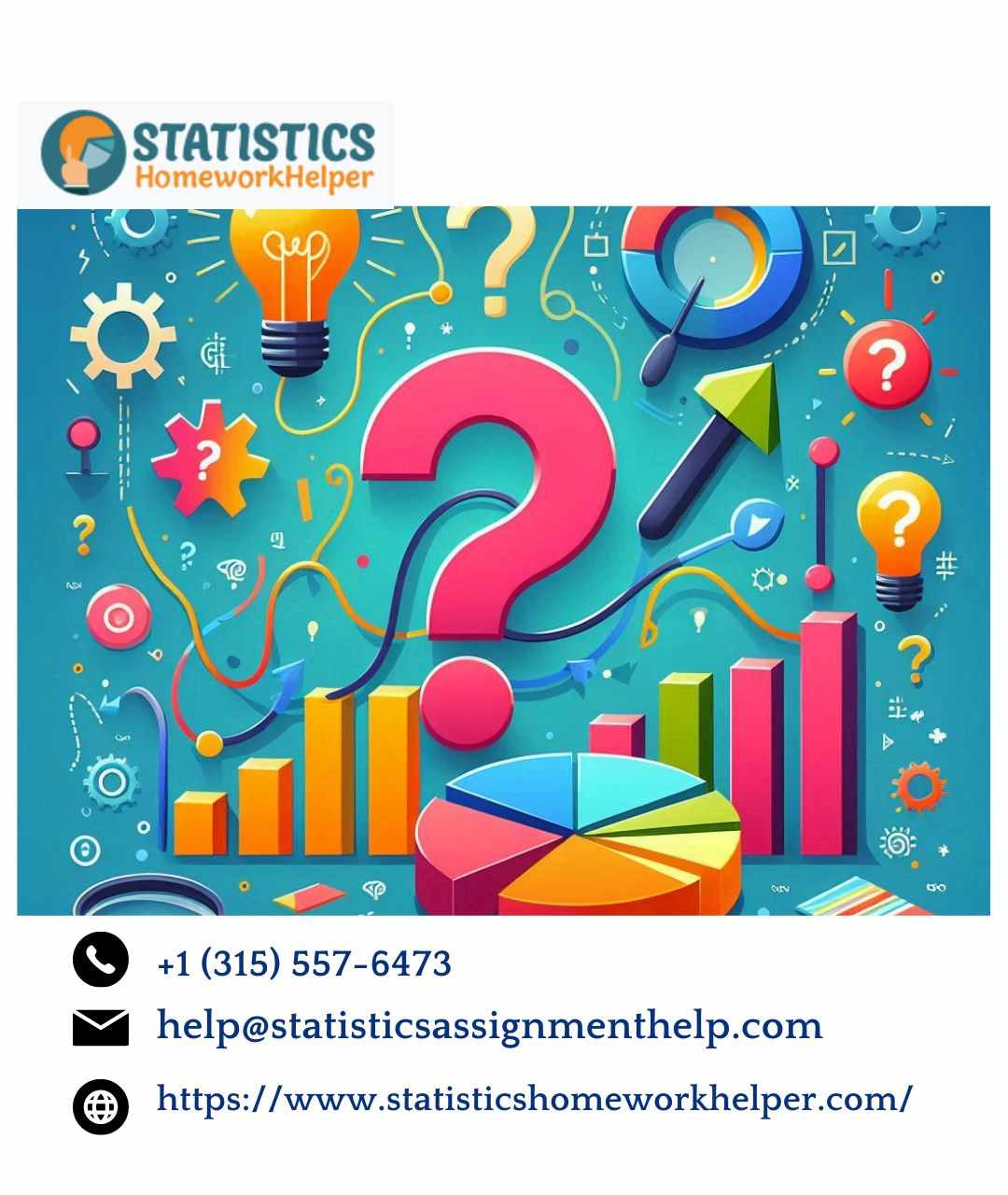
In the world of advanced statistics, mastering complex concepts is crucial for success in academia and beyond. For those grappling with intricate topics, finding reliable help can be a game-changer. If you’re wondering, “Who will do my R programming homework?” you’re not alone. Many students struggle with advanced statistical questions that require a deeper understanding and expert guidance. In this blog, we will delve into two sophisticated statistical questions, offering clear and comprehensive answers that illustrate the level of expertise required to tackle such challenges.
Question 1:
Consider a research study that investigates the relationship between several independent variables and a single dependent variable. The independent variables include factors such as age, income, education level, and occupation, while the dependent variable is a measure of job satisfaction. How would you approach analyzing this multivariate regression model, and what considerations should be made to ensure the model's validity?
Answer:
When analyzing a multivariate regression model where the objective is to examine the relationship between multiple independent variables and a single dependent variable, several key steps and considerations must be taken into account:
Model Specification: Begin by specifying the model based on the theoretical framework and research questions. In this case, the model aims to understand how age, income, education level, and occupation influence job satisfaction. Ensure that the model includes all relevant variables and that they are correctly specified.
Data Preparation: Before fitting the model, it is essential to prepare the data. This includes checking for missing values, ensuring that the data is appropriately scaled, and performing exploratory data analysis to understand the distribution and relationships between variables.
Assumptions Checking: Multivariate regression models rely on several assumptions, including linearity, independence, homoscedasticity (constant variance of errors), and normality of residuals. Verify these assumptions using diagnostic plots and statistical tests. For example, residual plots can help identify issues with homoscedasticity.
Multicollinearity: Assess multicollinearity among the independent variables. High multicollinearity can lead to unreliable estimates of regression coefficients. Use variance inflation factors (VIF) to detect multicollinearity and consider removing or combining variables if necessary.
Model Fitting: Fit the regression model using appropriate software or statistical packages. Ensure that the model is properly estimated and that the coefficients are interpreted in the context of the research question. For instance, a positive coefficient for income might suggest that higher income is associated with higher job satisfaction.
Interpretation and Validation: After fitting the model, interpret the results carefully. Evaluate the significance of each independent variable using p-values and confidence intervals. Additionally, assess the model's overall fit using metrics such as R-squared and adjusted R-squared. Validate the model using techniques such as cross-validation to ensure its generalizability.
Report Findings: Clearly report the findings, including the significance and direction of the relationships between independent variables and job satisfaction. Discuss any potential limitations of the model and suggest areas for further research.
By following these steps, you can ensure a rigorous analysis of the multivariate regression model, leading to valid and reliable conclusions about the factors influencing job satisfaction.
Question 2:
In psychometrics, factor analysis is commonly used to identify underlying constructs measured by a set of observed variables. Suppose you have a dataset with multiple psychological scales, and you want to determine the underlying factors that explain the observed correlations between these scales. What approach would you take to conduct factor analysis, and how would you interpret the results?
Answer:
Factor analysis is a powerful statistical technique used to uncover latent variables or constructs that explain the relationships between observed variables. When conducting factor analysis in psychometrics, the following approach can be used:
Determine the Objective: Define the objective of the factor analysis. In this case, the goal is to identify the underlying factors that explain the correlations among psychological scales. Clarify the theoretical constructs that the factors are expected to represent.
Select the Method: Choose between exploratory factor analysis (EFA) and confirmatory factor analysis (CFA). EFA is used when the underlying factor structure is unknown, while CFA is used to test a hypothesized factor structure. For initial exploration, EFA is typically employed.
Assess Data Suitability: Check the suitability of the data for factor analysis. This involves evaluating sample size, checking for correlation between variables, and ensuring that the data meets the assumptions of factor analysis. Use measures such as the Kaiser-Meyer-Olkin (KMO) test and Bartlett’s test of sphericity to assess the adequacy of the data.
Extract Factors: Perform factor extraction using techniques such as principal component analysis (PCA) or common factor analysis. Determine the number of factors to retain based on criteria such as eigenvalues greater than 1, scree plots, and theoretical considerations.
Rotate Factors: Apply factor rotation (e.g., varimax or oblimin) to achieve a more interpretable factor structure. Rotation helps to simplify the factor loadings and clarify the relationship between factors and variables.
Interpret Results: Examine the factor loadings to understand which variables are associated with each factor. High loadings indicate strong relationships between variables and factors. Interpret the factors based on the observed patterns and the theoretical constructs they represent.
Validate and Refine: Validate the factor structure by assessing model fit using indices such as the chi-square test, comparative fit index (CFI), and root mean square error of approximation (RMSEA). Refine the factor model if necessary, based on fit indices and theoretical considerations.
Report Findings: Present the results of the factor analysis, including the number of factors identified, their loadings, and their interpretative labels. Discuss how the factors contribute to understanding the underlying constructs measured by the psychological scales.
By following these steps, you can effectively use factor analysis to uncover and interpret the latent constructs underlying your data, providing valuable insights into the psychological phenomena being studied.
Conclusion
Mastering advanced statistical concepts and techniques is essential for success in higher-level academic research. By addressing complex questions with detailed and methodical approaches, students can enhance their understanding, refine their analytical skills, and improve their research outcomes significantly. Effective problem-solving, thorough analysis, and expert guidance pave the way to achieving academic excellence and success in their statistical endeavors. Developing a deep grasp of these advanced methods not only boosts academic performance but also prepares students for professional challenges and contributions in their field.
Write a comment ...